Working Group Webinar Library
Webinar Library
Prevails and Mirages of Large Language Models in Clinical NLP
This talk will introduce the technologies powering LLMs, overview the recent prevails, and examine the mirages in the hype of LLM magic. Based on the experience in developing two clinical LLMs in the clinical domain, including GatorTron and GatorTronGPT, this talk will provide insight into the potential application of LLMs for clinical NLP and healthcare.
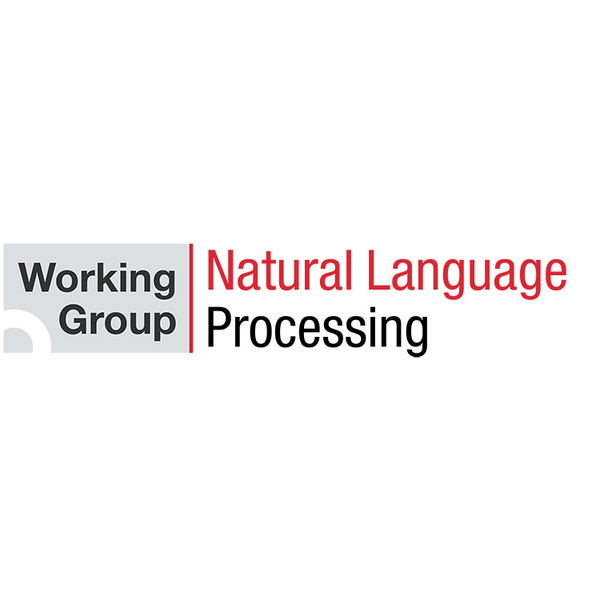
Reducing Diagnostic Delays in Acute Hepatic Porphyria Using Health Records Data and Machine Learning
Acute hepatic porphyria (AHP) is a rare but treatable condition with an average diagnostic delay of 15 years. Utilizing electronic health records (EHR) data and machine learning (ML) can potentially improve the timely recognition of AHP. This study used structured and notes-based EHR data from UCSF and UCLA to develop models predicting who will be referred for AHP testing and who will test positive. The referral model achieved an F-score of 86%-91%, and the diagnosis model achieved an F-score of 92%.
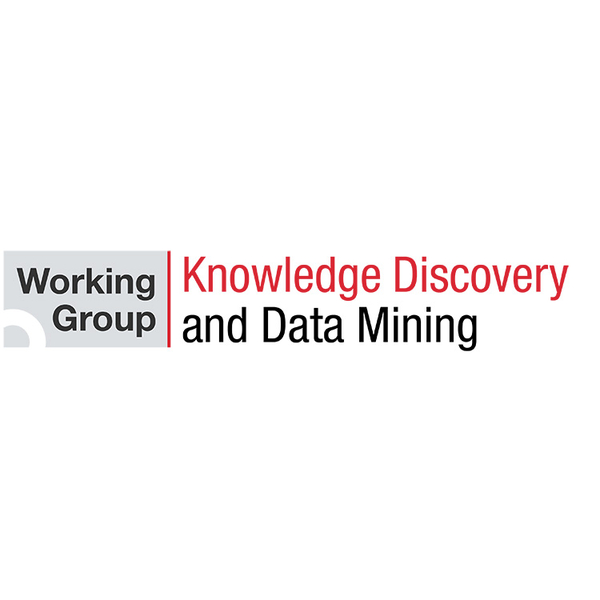
Translational Artificial Intelligence in Advancing Learning Health System (LHS) Systems
Learning health system (LHS) aims to leverage technology, data analytics, and evidence-based practices to create a feedback loop that continuously informs healthcare delivery, policy, and practice. It requires a multidisciplinary approach to interpret patterns observed in real-world data with the associated context.
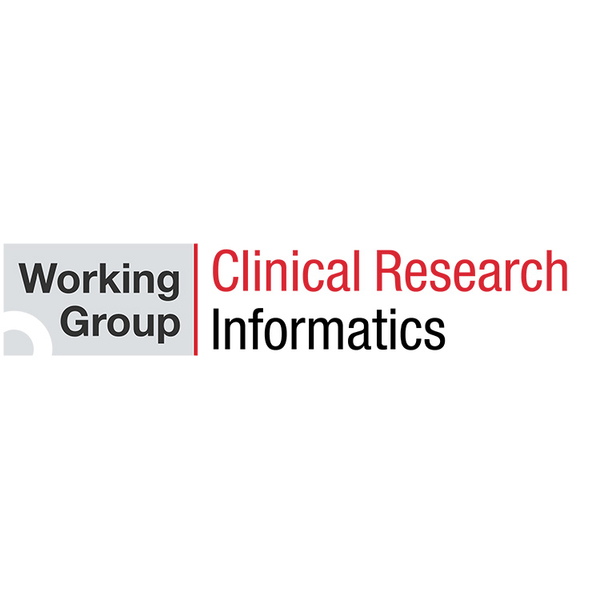
Implementation of Custom Prediction Models in the EHR - A Case Study on Postpartum Depression
This study describes the deployment process of an AI-driven clinical decision support (CDS) system to support postpartum depression (PPD) prevention, diagnosis and management. Central to this CDS is a predictive model trained on electronic health record (EHR) data at an academic medical center, and subsequently refined through a broader dataset from a consortium to ensure its generalizability and fairness.
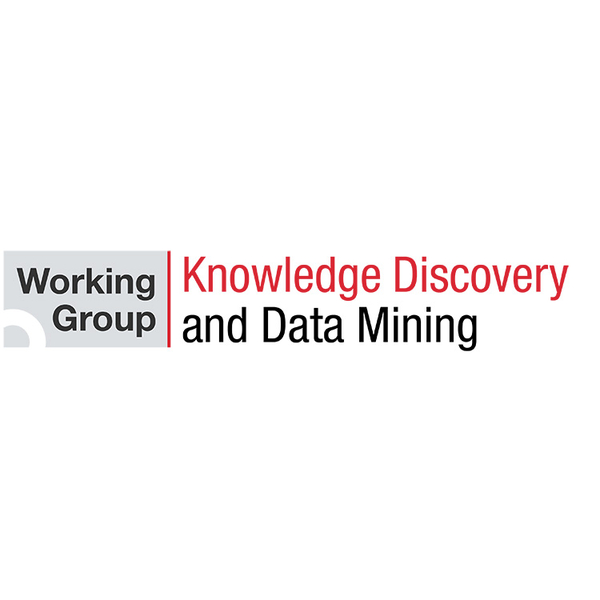
Leveraging Electronic Health Record Data and Natural Language Processing and Machine Learning for Early Detection of Pancreatic Cancer
Pancreatic cancer (PC) is ranked as the 11th most common cancer in the world with 458,918 new cases in 2018. It is projected to be the second leading cause of cancer-related mortality in the United States by 2030. Most of the mortality is attributed to advanced stage at diagnosis, and hence, only a minority of patients (15-20%) are eligible for surgical resection.
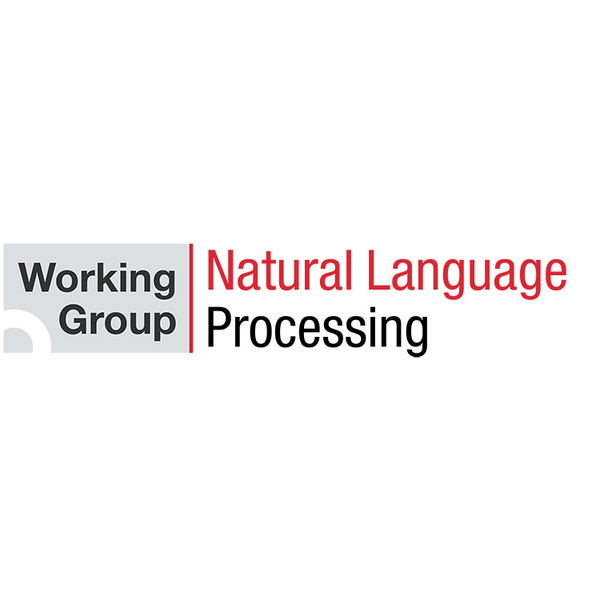